Prediction of tumoral movements during Free breathing for radiotherapy
Winter 2022: Emilie Ouraou (Master's)
Radiation therapy is a type of treatment against cancer. It aims at destroying all of the patient’s cancerous cells while limiting as much as possible the destruction of normal cells nearby so as to minimize side effects. Consequently this process requires to precisely identify the cancerous cells. However those cells move due to patient’s respiration, especially lungs tumors, thus reducing the precision of the treatment.
The goal of this project is to construct a model capable of predicting the movement of lungs tumor during the respiratory cycle in order to obtain a better precision in radiation therapy.
Noninvasive Characterization of The Tumor Immune Microenvironment of Colorectal Liver Metastases: A Multiomics Approach
Winter 2021: Ralph Saber (Doctorate)
In an effort to improve the understanding of tumor biology in colorectal cancer (CRC), it was found that CD3+ tumor infiltrating lymphocytes (TIL) had a strong prognostic value in primary CRC as well as in colorectal liver metastases (CLM). However, quantification of TILs remains labor intensive and requires tissue samples. In this study, we propose a radiomics-based pipeline to predict CD3 T-cell infiltration status in CLM from pre-operative computed tomography (CT) images. Our findings demonstrate a relationship between CT radiomic features and CD3 tumor infiltration status with the potential of noninvasively determining CD3 status from pre-operative CT images.
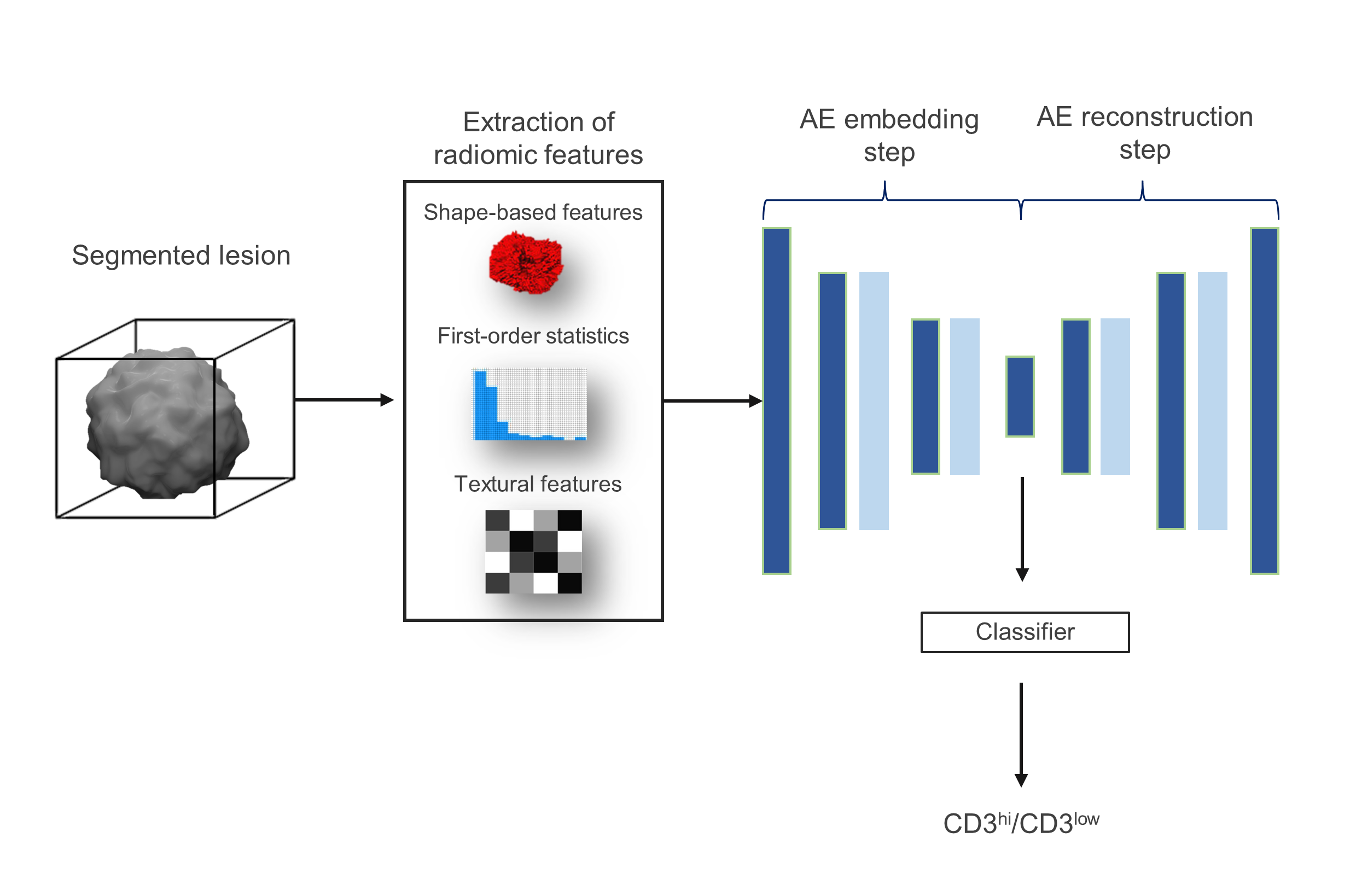
Submission: SPIE Medical Imaging 2022
Semi-supervised tumor response grade classification from histology images of colorectal liver metastases
Fall 2020: Mohamed Amine Elforaici (Master's)
Colorectal liver metastases (CLM) develop in almost half of patients with colon cancer. Response to systemic chemotherapy is the main determinant of patient survival. Due to the importance of assessing treatment response of CLM to chemotherapy for the patient prognosis, there is a need to classify tumor response grade (TRG) on histopathology slides (HPS). However, annotating HPS for training neural networks is a time-consuming task. In this work, we present an end-to-end approach for tissue classification of CLM slides leading to TRG prediction. A weakly-supervised model is first trained to perform tissue classification from sparse annotations, generating segmentation maps. Then, using features extracted for these maps, a secondary model is trained to perform the TRG classification. We demonstrate the feasibility of the proposed approach on a clinical dataset of 1450 HPS from 232 CLM patients by comparing our semi-supervised Mean Teacher approach with other supervised and semi-supervised methods. The proposed pipeline outperforms other models, achieving a classification accuracy of 94.4%. Based on the generated classification maps, the model is able to stratify patients into two TRG classes (1-2 vs 3-5) with an accuracy of 86.2%.
Submission: ISIBI 2022
Alzheimer’s disease (AD) as a most general cause of degenerative dementia is characterized by insidious onset, early memory loss, language and visuospatial deficits (associated with the destruction of the temporal and parietal lobes), a progressive course, and lack of early neurological signs early in the course of disease. Accurate differential diagnosis and cognitive impairment require an integrated patient’s observation. Different types of dementia are diagnosed by cognitive screening which is usually sensitive to significant cognitive impairment associated with dementia, and in some patients can also detect mild cognitive impairment (MCI). From a neuroimaging point of view, positron emission tomography(PET) images of fluorodeoxyglucose (FDG) for cerebral glucose metabolism and amyloid plaque images (AV45) can provide complementary information for diagnosis of AD. This project aims to develop a diagnostic aid system to classify the AD patients versus mild cognitive impairment patients using PET images. The ability to diagnose AD at an early stage has great impact on the clinical intervention and treatment planning, and hence reduced costs associated with the long term care. We are trying to show if it's possible to classify between AD, EMCI, LMCI and NC just using the PET images as main research question for this work. In this project, we are about finding out the best feature extractions from the FDG PET and AV45 PET images via some tools that’s already exist. Generally to have an accurate classification, the classifiers are important, looking up the most effective classifiers is another achievement in this project.
Alzheimer's disease (AD) is a neurodegenerative disease with symptom of dementia worsen over years. The ability to diagnose early onset of AD has great impact on treating AD and other dementias. Morphometric measures such as cortical thickness and tensor based shape descriptors from cortical and subcortical structures of brain have been widely used to charaterize AD and mild cognitive impairment (MCI) from normal cognitive ((NC) subjects. Common approaches involve finding a low-dimensional representation of these complex and high-dimensional data using principal component analysis (PCA) and multidimensional scaling (MDS). However these methods typically works in linear space and hardly able to process irregular or abnormal structures lying in nonlinear space. Manifold learning methods on the other hand is well known for its capability to model data in nonlinear space. This project aims to develop a new manifold learning framework for better charaterization of subjects with different stage of cognitive function, from cognitive normal (CN) to mild cognitive impairment (MCI) and AD. Through the exploration of high-dimensional neuroimaging data in a topologically invariant manifold learning framework, we anticipate to learn the brain development trajectory and efficiently combine multimodal and temporal data from MR and PET images for differentiating inter-subject brain variation among CN, MCI and AD subjects.
Adolescent idiopathic scoliosis (AIS) refers to a complex deformation in three dimensions (3D) of the spine. Classification of the rich and complex variability of spinal deformities is critical for comparisons between treatments and for long-term patient follow-ups. AIS characterization and treatment recommendations currently rely on the Lenke classification system, a two-dimensional (2D) assessment of scoliosis from radiographs of the spine in the coronal and sagittal plane. However, misinterpretations could arise because two different scoliosis deformities may have similar 2D parameters. The objective of this project is to develop computational methods that will capture the intrinsic dimensionality of 3D spine models. The highly dimensional 3D spine models are mapped into a manifold of much lower dimensionality. A clustering method is then performed to find sub-groups within the studied population. Identifying groups based on their true 3D representation will ensure a better understanding and description of the curve morphology. Moreover, this will help adjusting surgery choices such as levels of fusion, biomechanical forces to apply or surgical instrumentations.
Adolescent idiopathic scoliosis (AIS) is a 3D deformity of the spine. However, the most common methods of evaluating this deformity rely on two-dimensional measurements on x-rays. Consequently, these descriptors do not adequately describe this deformity in 3D. Geometric torsion is a true 3D descriptor of scoliosis and measures a curves tendency to twist. It has previously been studied in AIS, but due to the local approach taken, the measurement is highly susceptible to errors in 3D reconstructions of scoliosis. The objective of this study is to evaluate geometric torsion in AIS using a global approach in estimating torsion values that employs local arc-length approximations. By numerically quantifying torsion levels, this project ultimately aims to present an intuitive and user friendly 3D descriptor of scoliosis as well as classification scheme.
Automatic treatment outcome prediction is an active area of research in medical image analysis. Accurate results could lead to adapted treatment planning and replanning during disease progression, while minimizing side-effects on patient quality of life. However, while most modern machine learning techniques propose generalizability across individuals, the same is not true across medical institutions. Indeed, differences in scanner manufacturers, models, parameters and operators can all lead to significant differences in collected diagnostic images which impact existing machine learning based classifier performance. Comparatively, traditional diagnosis methods by domain expert involve visual analysis of medical images; while humans are easily capable of ignoring multi-domain differences, they are limited to visual imaging information, whereas automatic methods often present the opposite effects. New research is therefore required to combine both advantages using modern deep learning methods, which have been shown to excel in natural image analysis. In this work we thus propose a CNN based approach that leverages multi-institutional and multi-modal head and neck cancer dataset to predict 10-year survival outcomes as well as tumor regression.
